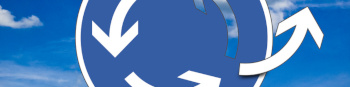
Evolving GraphQL Schemas in Spring Boot: Best Practices
GraphQL APIs are designed to evolve continuously without requiring explicit version increments. Unlike REST, where introducing breaking changes often means creating a new versioned endpoint, GraphQL encourages a single versionless API that grows over time. This is possible because clients only retrieve fields they ask for, so adding new capabilities doesn’t break existing queries. For example, you can introduce new fields or types in the schema and old clients won’t be affected since they won’t request those new fields. The goal is to avoid breaking changes whenever possible and use GraphQL’s built-in tools (like deprecation) to guide clients through changes. This agile, incremental approach to schema development is even highlighted in GraphQL best practices (Principle #5: “the schema should … evolve smoothly over time”).